A paper published in Nature presents a machine learning model capable of producing reliable probabilistic weather forecasts. The model, called GenCast, outperforms the most efficient traditional medium-range weather forecasting and is also able to better predict extreme weather, tropical cyclone tracks and wind energy production.
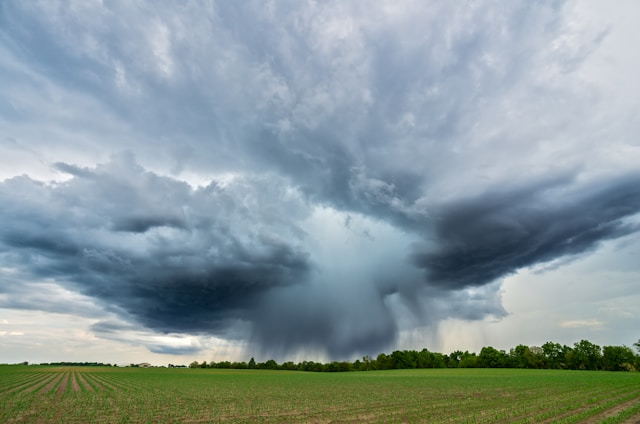
Ernesto Rodríguez - modelo
Ernesto Rodríguez Camino
Senior State Meteorologist and member of Spanish Meteorological Association
The GenCast prediction system is an evolution of GraphCast, developed by Google DeepMind, using machine learning (ML) techniques based on data, as opposed to traditional numerical methods grounded in the laws of physics.
In recent years, major tech companies, such as Google, have been advancing the quality of weather predictions with their respective models based solely on machine learning, to the point of surpassing the skill of the IFS model from ECMWF (European Centre for Medium-Range Weather Forecasts), which is considered the gold standard for medium-range prediction quality. Until now, machine learning-based prediction systems had focused on deterministic forecasts. However, this article reports a significant step forward with the development of probabilistic forecasts, based on an ensemble of predictions generated from slightly different initial data. This approach has also demonstrated the ability to outperform the ECMWF's ensemble prediction system while showcasing great skill in predicting extreme weather events.
Nevertheless, systems like the GenCast described here rely on reanalysis obtained through traditional methods based on physical laws. As such, it remains dependent on the ECMWF's IFS model, both to establish initial conditions and to train machine learning algorithms. Additionally, GenCast is highly efficient in computational terms, paving the way for the foreseeable future development of hybrid assimilation and prediction systems. These systems could leverage the strengths of both approaches: physical equation-based models to validate, train, and improve the system, and data-driven models to optimize prediction calculations and enhance ensemble prediction resources.
- Research article
- Peer reviewed
Price et al.
- Research article
- Peer reviewed