An artificial intelligence (AI) model led by the company Meta is capable of translating speech and text, including direct speech-to-speech translations, from up to 101 languages in some cases. According to the research team, this model - called SEAMLESSM4T - can pave the way for fast universal translations ‘with resources to be made publicly available for non-commercial use’. The work is published in the journal Nature.
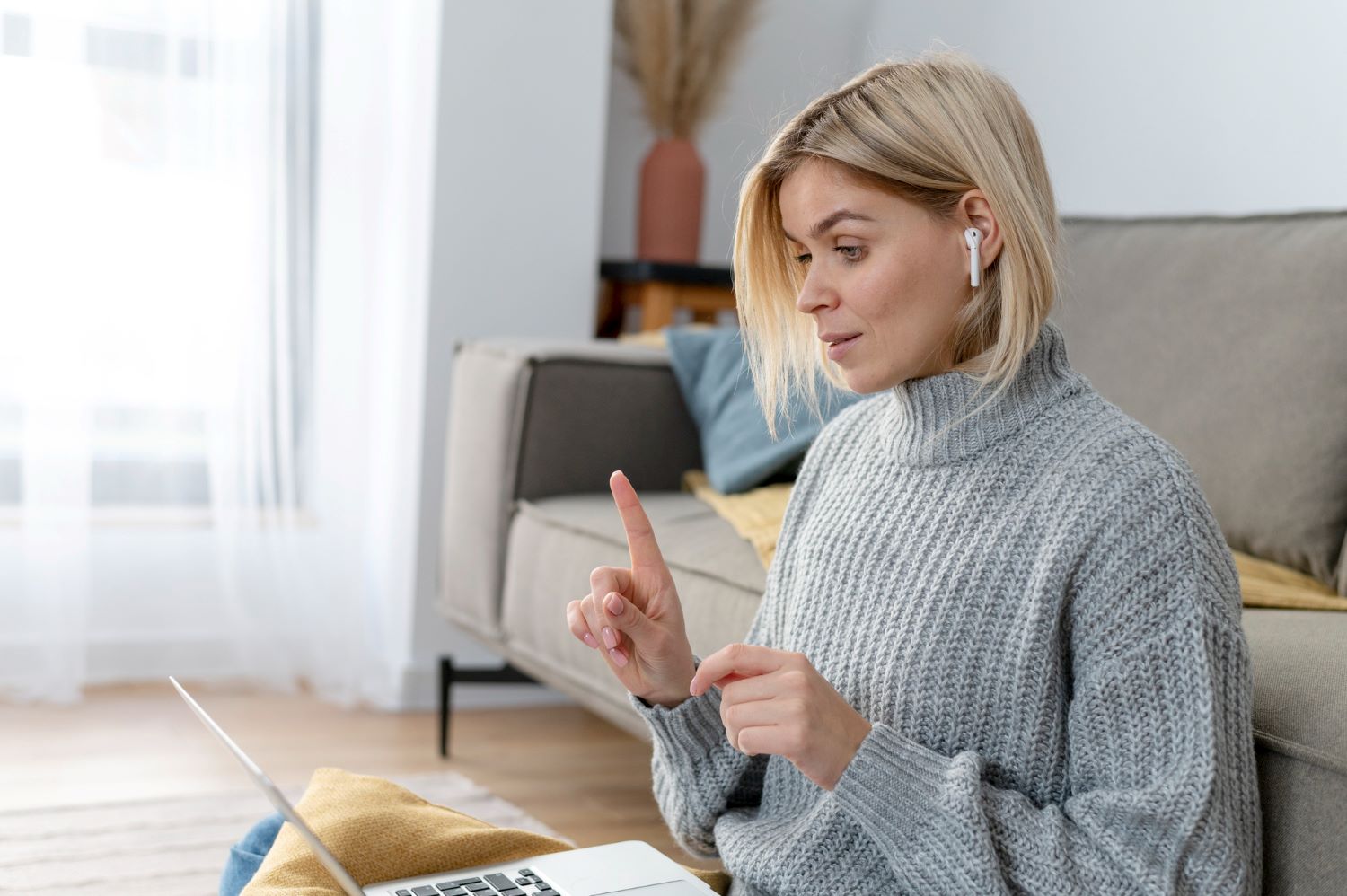
Víctor Etxebarria - IA Meta voz EN
Víctor Etxebarria
Professor of Systems Engineering and Automatics at the University of the Basque Country (UPV/EHU)
The SEAMLESSM4T translator presented by the company Meta is an advanced technological product that brings together technologies previously developed by many people involved in AI R&D. The article published in Nature -Joint speech and text machine translation for up to 100 languages- does not contribute to scientific progress. The article published in Nature -Joint speech and text machine translation for up to 100 languages- does not contribute to scientific progress, since, on the basis of what has been published, independent specialists are not allowed to reproduce, test or even improve their technological bases, and only have access to connect to the translator to perform superficial examples of translations. This software does not comply with the principles of open source AI as defined by the Open Source Initiative: use, study, modify and share for any purpose. This is not allowed by this translator and is therefore not consistent with the principles of open science.
The translator, especially in its version of direct speech-to-speech translation, is a product that can be very useful, trying to mimic the service performed by simultaneous translators in the international context. The product does not prevent translation delays, nor does it prevent translation errors, nor does it prevent their correction in real time, which is done by translators. Another limitation is that it can only be used through the remote API (Application Programming Interface) via the internet, which is imposed by the company. All in all, the translator is a technologically advanced and probably very useful product, but closed to the principles of open science and with multiple technological and legal limitations.
Maite Martín - IA Meta voz EN
Maite Martín
Professor of the Department of Computer Science at the University of Jaén and researcher of the research group SINAI (Intelligent Systems for Information Access)
The paper presents a multimodal, multilingual machine translation model called SEAMLESSM4T, developed to overcome current limitations in text-to-speech translation, including translations between resource-poor languages. This unified model enables tasks such as speech-to-speech, speech-to-text, text-to-text and text-to-speech translation, with support for up to 101 source languages and up to 36 target languages in speech modalities.
In my view, one of the highlights of the model is its focus on studying and incorporating under-resourced languages, such as Maltese and Swahili, which have historically been excluded from technological advances in machine translation. These languages, lacking large volumes of tagged data and specific resources, are often left behind in the development of advanced linguistic tools. However, the work addresses this gap by creating a massive corpus of aligned speech and text data. This corpus combines manually tagged data with automatically generated resources, which significantly extends the scope and accuracy of the model in under-represented languages. This effort not only improves the accessibility of translation technologies for these communities, but also marks an advance in linguistic inclusion by democratising access to advanced communication tools.
An equally relevant aspect of the work is the decision to make these data and tools available to the scientific community for non-commercial use. This approach fosters collaborative research by allowing other developers and researchers to use these resources to further advance machine translation, especially in multilingual and multimodal contexts. The publication of these resources not only consolidates the model as a benchmark in technological innovation, but also drives the development of more inclusive and equitable solutions, laying the foundations for a more open and dynamic research ecosystem.
The model, however, also faces important limitations. Although it improves translation accuracy in resource-poor languages, the results are still inferior to those obtained with high-availability languages. In addition, aspects such as real-time interaction, expressiveness of the translated speech, and mitigation of gender bias and toxicity remain open challenges. These limitations suggest that, although SEAMLESSM4T represents a significant advance, there is still work to be done to optimise its implementation in practical scenarios.
Andreas Kaltenbrunner - IA Meta voz EN
Andreas Kaltenbrunner
Lead researcher of the AI and Data for Society group at the UOC
This is a very interesting study, although not so recent. Meta already published a first version of the study in August 2023. Despite this, this study incorporates several notable innovations.
Firstly, it is a unified system that manages all aspects of translation (speech and text) in a single environment, instead of relying on several independent systems.
Another relevant aspect is the large number of languages it supports: more than 100 input languages and dozens of output languages. It also stands out for its robustness in the face of real-world challenges, such as handling noise and understanding different accents, aspects that often cause difficulties for other systems.
In terms of performance, it outperforms the best previous systems on a number of metrics, with an improvement of more than 20%.
Finally, it is commendable that the study includes an analysis of whether translations increase the toxicity of texts or how they address possible gender bias. However, it is unfortunate that Meta, the employer of the researchers in this study, seems to have recently decided to abandon efforts in this regard with its new content moderation policy.
Pablo Haya - IA Meta voz EN
Pablo Haya Coll
Researcher at the Computer Linguistics Laboratory of the Autonomous University of Madrid (UAM) and director of Business & Language Analytics (BLA) of the Institute of Knowledge Engineering (IIC)
SEAMLESSM4T is a multilingual, multimodal machine translation system that combines speech-to-speech (S2ST), speech-to-text (S2TT), text-to-speech (T2ST) and text-to-text (T2TT) translation capabilities for a very wide range of languages, including resource-poor languages. SEAMLESSM4T achieves higher accuracy and robustness than traditional translation systems. Reported metrics indicate that the model is resistant to noise and speaker variation.
Interestingly, the model incorporates strategies to mitigate gender bias and toxicity, ensuring more inclusive and safer translations. SEAMLESSM4T represents a step forward in building inclusive and accessible systems, offering an effective bridge between cultures and languages for application in both digital and face-to-face contexts.
While SEAMLESSM4T is a significant advance, it has some notable limitations. Its success varies by language, especially in low-resource languages, and by gender, accent and demographics. It may face difficulties in translating proper names, slang and colloquial expressions.
It should be borne in mind that speech is not limited to being spoken text; it incorporates a variety of prosodic components, such as rhythm, stress, intonation and tone, as well as emotional elements that require further investigation. In order to develop S2ST systems that are organic and natural, it is essential to focus efforts on ensuring that the audio generated preserves the expressiveness of the language.
Furthermore, to increase the adoption of these systems, more research is needed on systems that allow for streaming translation, i.e. incrementally translating a sentence as it is spoken.
Finally, the authors themselves stress that SEAMLESSM4T-driven applications should be understood as support tools designed to assist translation, rather than replacing the need for language learning or reliable human interpreters. This reminder is especially crucial in contexts such as legal or medical decision-making.
Author's note: ‘SEAMLESSM4T was published openly by Meta in August 2023. The paper published in Nature does not seem to differ from what Meta has already explained and made openly available in its github repository. It is possible to test this technology here.'
Raquel Fernández - IA Meta voz EN
Raquel Fernández
Full Professor of Computational Linguistics & Dialogue Systems at the University of Amsterdam and the Vice-Director for Research at the Institute for Logic, Language & Computation (ILLC)
The article presents a single model able to translate from written text to written text, from speech to speech, and from text to speech or speech to text between around 100 different languages. Automatic machine translation systems tend to work reasonably well for languages that are well represented online (English, Chinese or Spanish, etc.) but less so for languages with fewer speakers or less available digital data (such as Maltese, Swahili, or Urdu). Moreover, automatic translation has mostly been confined to written text. The model presented in this article (SEAMLESSM4T) advances the field by including the ability to translate to and from speech (in addition to text) and by doing so for a large quantity of different languages.
As all current AI systems, automatic machine translation models require huge amounts of data for training. In the case of translation, the data typically consists of pairs of sentences: a sentence in a given language and its translation to another language, which the model uses to learn. However, this kind of paired data is very costly to create and not available for many languages. The authors address this problem by using an AI model (SONAR) able to find sentences online (written or spoken) that have very similar meanings and use these mined sentences as a proxy for translation pairs. This allows them to create a very large training dataset that is key for developing a robust translation model. Beyond offering more coverage than earlier models, the resulting model tends to lead to translations that are of higher quality regarding meaning, sound, and clarity.
While this model makes substantial progress on speech translation, translating into spoken language remains more challenging than outputting text: the model can output translated text in 96 languages, but currently it can produce a spoken translation in only 36 languages. Moreover, the automatically produced speech may not always be expressive and sound natural. Similarly, when translating from speech to text, the model may have trouble processing the speech based on factors such as gender, accent, or language. An evaluation of the model’s performance also shows that it tends to display gender bias, for example when a sentence in the source language does not specify the gender (as in the English sentence “I’m a homemaker”, where the person speaking may be of any gender), the model has higher tendency to output a certain stereotypical gender when translating into gendered languages (e.g., when translating into Spanish from English, it may have a higher tendency to produce “Soy ama de casa” than “Soy amo de casa”). Finally, while the model has the potential to improve communication in many everyday scenarios, it does not yet allow for streaming or simultaneous translation, that is, the translation of a sentence as it is being produced.
Rocío Romero - IA Meta voz EN
Rocío Romero Zaliz
Full Professor of the Department of Computer Science and Artificial Intelligence at the University of Granada
Machine translation has evolved from rule-based systems and statistical calculations to today's large linguistic models or LLMs, thanks to the computational power available. Within this context, the publication presents a breakthrough in faster, more reliable and universal translation systems. It highlights the ability to perform speech-to-speech translations directly, without intermediate steps (speech-to-text, text-to-text, text-to-speech translation), speeding up the process. In addition, it supports multiple languages, bringing us ever closer to the utopia of a universal automatic translator. It is also interesting to note how the improvements discussed in the publication are not based on a greater number of parameters of the model to be trained, but on a more intelligent preprocessing of the available information, even incorporating new sources of additional information to improve translations.
So far, most machine translators translate from language X to Y using English as an intermediary. However, this publication proposes direct translations from language X to language Y, thus eliminating accumulated errors. This is achieved through the use of a common representation space where sentences with similar meanings are close together, regardless of language. However, the training used is still largely based on translations from or into English. Moreover, all the tests and trials shown in the main text of the publication have been carried out between language X and English, or vice versa. It will then be necessary to review the supplementary material in the publication and to test the proposed system once available between language pairs that do not include English or some other major language, which remains a challenge. Finally, it should be noted that, although the speech-to-speech translation is performed correctly, it does not take into account vocal inflections and other emotional components that may affect the accuracy of the final translation.
Rodolfo Zevallos - IA Meta traductor EN
Rodolfo Zevallos
Researcher in the Language Technologies group at the BSC (Barcelona Supercomputing Center)
The article ‘Joint Speech and Text Machine Translation for up to 100 Languages’ presents SEAMLESSM4T, a multilingual machine translation model that marks a major breakthrough in the field by unifying multiple tasks into a single, robust and efficient system. It supports a wide range of functions, including automatic speech recognition (ASR), text-to-text (T2TT), text-to-speech (T2ST), speech-to-text (S2TT) and speech-to-speech (S2ST), all in a number of languages. It is also notable for its modular design, which allows each component to be used independently. This flexibility is particularly valuable, as it facilitates customisation, optimises the use of resources and enhances its applicability in a variety of practical contexts.
The performance of the model is excellent compared to the state of the art. Moreover, the model's robustness to background noise and speaker variability is another positive aspect, ensuring a high level of accuracy even under adverse conditions. Its contribution to a more responsible artificial intelligence is also remarkable, with significant reductions in toxicity levels and a systematic assessment of gender bias, essential aspects to ensure fairness in its use.
Finally, given the level of innovation and technical complexity of the model presented in the paper, it would be beneficial to have a more extensive version of the article, which would allow us to explore in greater detail the methodological and technical aspects that underpin it. In addition, it would be interesting to further explore the tokenisation (word segmentation) process, particularly for morphologically complex languages, where an adequate representation is crucial to improve the quality of translations.
- Research article
- Peer reviewed
SEAMLESS Communication Team
- Research article
- Peer reviewed